Face recognition is mainly divided into four processes: face detection, image preprocessing, feature extraction and matching recognition (as shown in Figure 1).
Face detection
Face detection is mainly used for face recognition preprocessing in practical applications, that is, the face image is detected and extracted from the input image, and the position and size of the face are calibrated. The commonly used face image pattern features include: histogram features, color features, structural features, and haar features. Based on the above features, the Adaboost algorithm is used to select the rectangular features that best represent the human face. In accordance with the weighted voting method, a cascaded classifier is constructed in stages. At the time of detection, the cascaded classifier classifies each block in the image and finally determines the face image through the image of the cascaded classifier.
Figure 1 Face Recognition Process
2. Image preprocessing
The original image acquired by face detection is often not directly used due to the constraints of various conditions and random interference. It is necessary to include the light compensation, gray scale transformation, histogram equalization, and normalization of the image in the face image preprocessing section. , filtering and sharpening.
Feature extraction
Feature extraction is the most critical step in face recognition. Simply speaking, it means that face information is represented by some numbers. These figures are the features to be extracted.
The current mainstream feature extraction algorithms are mainly divided into linear feature extraction algorithms and nonlinear feature extraction algorithms. The linear feature extraction algorithm is represented by Principal Component Analysis (PCA) and Linear Discriminant Analysis (LDA).
Principal component analysis is a basic multidimensional data description method. Its basic idea is to use a set of few features to represent the features of the sample as accurately as possible. Principal component analysis usually uses the eigenvectors of the sample population covariance matrix as the expansion base (ie KL axis), and those eigenvectors corresponding to several largest eigenvalues ​​are called principal components or principal components. After the pattern samples are linearly projected on these principal components, the resulting coefficients are called principal component features. The principal component analysis has two major advantages: eliminating the correlation between pattern samples and achieving dimension compression of the pattern samples. That is, the principal component analysis gives a simple representation of the original high-dimensional samples. It can be shown that this representation is optimal in the sense of minimum covariance. Due to these advantages, principal component analysis was successfully applied to face image representations. However, since this representation is aimed at the optimal reconstruction of all the samples, it is not necessarily the best description for describing the differences between the samples of different classes. In this sense, using the features it describes for face recognition is not optimal.
Linear discriminant analysis is one of the most classical methods of many pattern recognition methods. The purpose of the LDA algorithm is to determine a set of optimal discriminant vectors (projection axes) so that the ratio of determinants between the class-to-class dispersion and intra-class dispersion is maximized after the original data is projected on the discriminant vector set, and the direction is said to correspond. The vector is Fisher's best discriminant vector. The physical meaning of LDA is that, after the samples are projected on these optimal discriminant vectors, the samples of the same kind are as close as possible, and the samples of different classes are separated as much as possible, and the ratio of the degree of inter-class dispersion to the degree of intra-class dispersion is maximized. If the PCA obtains the best representation feature set of the sample, then the LDA obtains the best set of discriminative features of the sample, which should be more suitable for pattern classification.
However, for face recognition, the distribution of face images in the high-dimensional space caused by the differences in facial expressions, light, and posture causes the distribution of face images to be nonlinear, and the linear feature extraction method is for these nonlinearities. The features are linearly simplified, so no better recognition results can be obtained. Therefore, the nonlinear feature extraction method has attracted researchers' extensive attention and has achieved great development. The nonlinear feature extraction method can be roughly divided into two branches, namely the kernel-based feature extraction method and the feature extraction method dominated by manifold learning.
4. Matching recognition
The extracted face features to be identified are compared with the face features in the database, and the face identity information is judged according to similarity. And this process can be divided into two major categories: one is one-on-one verification, one is one-to-many identification.
One-to-one verification refers to comparing the feature information of the person to be identified with the historical collection feature information. If the similarity between the two is not lower than the set threshold, the verification is passed, otherwise the failure occurs. In this model, the biometric technology performance is usually measured by using the FNR, False Non-Match Rate, and the False Match Rate (FMR, False Match Rate), as defined in (1) and (2). Show.
One-to-many identification is the use of unknown identity biometrics in a large number of known biometric database queries, set a similarity threshold, and return the length of the list to identify the identity of the owner of the unknown biometric. The recognition performance is generally measured by using the FPIR (False Positive Identification Rate) and the TPIR (True Positive Identification Rate). Specific definitions are shown in (3) and (4).
In the face recognition performance test, in order to measure the work intensity of manual viewing, the SEL (Selectivity) index is also defined to measure the performance of one-to-many recognition. The specific definition of SEL is shown in (5).
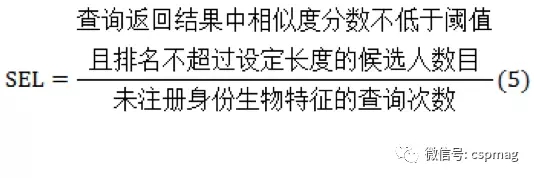
When the lighting conditions and face pose change (for example, if the face is deflected in the depth direction), the recognition rate of the face recognition system will be seriously reduced. In view of the above technical defects, in the field of face recognition technology, major universities and scientific research institutions are conducting more in-depth research, and a variety of new technologies and algorithms have emerged. For example, face recognition technology based on multiple clues introduces a variety of effective feature information and multiple theoretical reasoning methods in cognitive discrimination to fully and accurately recognize and distinguish objects; a robust face recognition algorithm based on linear representation, The algorithm first detects the noise points in the image, then obtains the accurate representation coefficient based on the noise removal, and makes a more accurate recognition. The feature extraction method of local maximum distance discrimination embedding (LMMDE) based on manifold learning is used. This method not only maintains the local structure of the sample, but also considers the differences of samples of different classes on the same manifold, effectively solving the problem of overlapping of different samples due to the distortion of the neighbor relationship.
Automatic Metal Baler
The trends of the Metal recycling industry
More than 5 million metric tons of aluminum are recovered each year. About 2 million metric tons of copper are recycled. Millions of tons more of other various metals are handled by the scrap metal industry as well. There is a lot of value in this metal, which is why it continues to be a strong industry, even in mature markets.
Two-thirds of the metals that the United Nations has identified as being available for recycling have a recovery rate that is below 50%. Although improving these rates would require waste management and infrastructure investments, the improved efficiency rates could offset many of those costs.
What is the role of Metal Baler in Metal recycling?
By baling the products, the volume of metal scrap is greatly reduced, often up to seventy-five per cent.
By reducing the volume in this way, the smelting costs are reduced. In addition, the overall process of recycling and re-casting can be done more quickly.
Automatic Metal balers are economically and environmentally beneficial in reducing metal waste. They lower costs, are space-efficient and make transporting the materials much easier.
The Automatic Metal Baling press makes your metal recycling more efficient and profitable.
How to choose a suitable metal baler?
The Y81 series Automatic Metal Scrap Baler could not only press all non-ferrous metals such as copper, brass, and aluminium can be recycled as well as ferrous metals from steel to stainless steel, with various sizes for option, high-density compression, compact and strong. Low energy consumption but fast production rate.
Y81 series hydraulic metal baler mainly include the following models.
Y81F (side turn discharge ), Y81T (side push discharge), Y81Q (front push discharge) and Y81S (Double main press ).
We also support the design and manufacture of other model versions upon request.
Y81Q-Manual operation (Front push discharge )
Y81F-Manual operation (Side turn discharge )
Y81F-Semi-automatic operation (Side turn discharge )
Y81T-Semi-automatic operation (Side push discharge )
Y81S-Semi-automatic operation (Double main press discharge )
We provide a variety of models of the hydraulic metal baler, With new technologies to raise the quality and reduce the price, which supports 24 hours fully automatic working environment.
YMSRECYCLING offer economical and effective solutions to help you to process different metal scraps.
The Metal Baling Press can be customized and extended according to the actual needs of your on-site.
CONTACT YMSRECYCLING
Call us now on +86-15950122559
To start maximising your returns from your metal scrap recycling.
Please email sales@ymsrecycling.com or visit our website directly for further information about the products you are interested in.
Automatic Metal Baler,Automatic Metal Scrap Baler,Automatic Metal Baling Press,Automatic Metal Scrap Baler
Jiangyin Yimaisheng Hydraulic Machinery Co., Ltd. , https://www.ymsrecycling.com